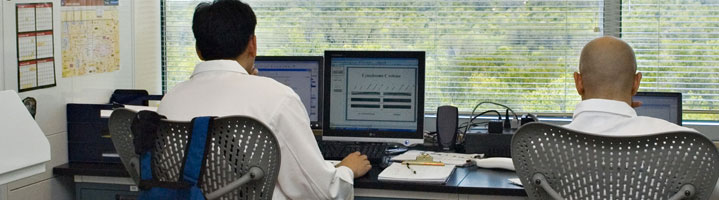
Low Use of Opioid Risk Reduction Strategies in Primary Care Even for High Risk Patients with Chronic Pain
JGIM ORIGINAL RESEARCH Low Use of Opioid Risk Reduction Strategies in Primary Care Even for High Risk Patients with Chronic Pain Joanna L. Starrels, MD, MS1, William C. Becker, MD2, Mark G. Weiner, MD3, Xuan Li, MS1, Moonseong Heo, PhD1, and Barbara J. Turner, MD, MSEd4 1Division of General Internal Medicine, Albert Einstein College of Medicine and Montefiore Medical Center, Bronx, NY, USA; 2Yale University School of Medicine, New Haven, CT, USA; 3University of Pennsylvania School of Medicine, Philadelphia, PA, USA; 4University of Texas Health Science Center and University Health System, San Antonio, TX, USA. BACKGROUND/OBJECTIVE: Experts recommend close oversight of patients receiving opioid analgesics for chronic non-cancer pain (CNCP), especially those at increased risk of misuse. We hypothesized that physicians employ opioid risk reduction strategies more frequently in higher risk patients. DESIGN: Retrospective cohort using electronic medical records. PARTICIPANTS: Patients on long-term opioids (=3 monthly prescriptions in 6 months) treated for CNCP in eight primary care practices. METHODS: We examined three risk reduction strategies: (1) any urine drug test; (2) regular office visits (at least once per 6 months and within 30 days of modifying opioid treatment); and (3) restricted early refills (one or fewer opioid refills more than a week early). Risk factors for opioid misuse included: age <45 years old, drug or alcohol use disorder, tobacco use, or mental health disorder. Associations of risk factors with each outcome were assessed in non-linear mixed effects models adjusting for patient clustering within physicians, demographics and clinical factors. MAIN RESULTS: Of 1,612 patients, 8.0% had urine drug testing, 49.8% visited the office regularly, and 76.6% received restricted (one or fewer) early refills. Patient risk factors were: age <45 (29%), drug use disorder (7.6%), alcohol use disorder (4.5%), tobacco use (16.1%), and mental health disorder (48.4%). Adjusted odds ratios (AOR) of urine drug testing were significantly increased for patients with a drug use disorder (3.18; CI 1.94, 5.21) or a mental health disorder (1.73; CI 1.14, 2.65). However, the AOR for restricted early refills was significantly decreased for patients with a drug use disorder (0.56; CI 0.34, 0.92). After adjustment, no risk factor was significantly associated with regular office visits. An increasing number of risk factors was positively associated with urine drug testing (p<0.001), but negatively associated with restricted early refills (p=0.009). Electronic supplementary material The online version of this article (doi:10.1007/s11606-011-1648-2) contains supplementary material, which is available to authorized users. Received July 19, 2010 Revised December 17, 2010 Accepted January 6, 2011 Published online February 24, 2011 CONCLUSION: Primary care physicians’ adoption of opioid risk reduction strategies is limited, even among patients at increased risk of misuse. KEY WORDS: opioid misuse; chronic pain; urine drug testing. J Gen Intern Med 26(9):958–64 DOI: 10.1007/s11606-011-1648-2 © Society of General Internal Medicine 2011 INTRODUCTION With increased prescribing of opioid analgesics for chronic non-cancer pain (CNCP) in the past decade, opioid abuse, addiction, and overdose death have risen in parallel.1–5 In response, pain management experts, medical societies, and regulatory agencies have published guidelines for long-term opioid treatment aimed at reducing opioid misuse,6–9 the use of prescribed opioids other than as directed or indicated.10 Recommendations include urine drug testing, regular face-toface office visits to evaluate patients’ response to opioids and risk of misuse, and adhering to a pre-defined refill schedule (i.e., restricting refills of opioids prior to expiration of the previous prescription). Although strong evidence to support these recommendations is lacking,11,12 experts endorse their role especially for patients at high risk of opioid misuse.7–9,13 Several patient characteristics have been associated with an increased risk of opioid misuse in observational studies. Younger age has been consistently associated with opioid misuse,14–18 with more than 70% of opioid overdose deaths occurring in individuals under age 45.19 Patients with a history of drug abuse or dependence have an increased risk of prescription opioid abuse or dependence15 and up to six times greater risk of aberrant medication-related behaviors such as prescription forgery or obtaining opioids from lay per 14,17,20 21 sons.Alcohol use disorders,14,21 tobacco use,and mental health disorders 15,20–22 are also associated with an increased risk for opioid misuse. Because primary care is the dominant health care setting in which opioids are prescribed for CNCP, we studied indicators of quality of care in monitoring for potential opioid misuse among primary care patients treated with long-term opioids. Specifically, our study aimed to: (1) evaluate the frequency of urine drug testing, regular office visits, and restricted early refills; and (2) examine the association of patient risk factors for opioid JGIM Starrels et al.: Opioid Risk Reduction Strategies in Primary Care 959 misuse with receipt of each of these three strategies. We hypothesized that use of risk reduction strategies would be low, but would increase among patients with risk factors for misuse. METHODS This retrospective cohort study used administrative data from eight urban or suburban primary care practices within the University of Pennsylvania Health System. All practices share an electronic medical record containing data on patient demographics, clinical diagnoses, inpatient and outpatient encounters, prescribed medications, and laboratory tests. The University of Pennsylvania Institutional Review Board approved this project. SAS version 9.1 (SAS Institute Inc, Cary, NC) was used to conduct all analyses. Study Sample Within a study timeframe from 01 January 2004 to 30 April 2008, we identified eligible patients aged 18 and older who met the following criteria: 1) three or more completed visits to a study primary care practice; 2) long-term opioid treatment defined by three or more opioid prescriptions written at least 21 days apart within 6 months;23 and 3) an inpatient or outpatient ICD-9-CM diagnosis for musculoskeletal or neuropathic pain (available online). We excluded patients with cancer, except non-melanoma skin cancer or remote prostate cancer because of low risk of current cancer-related pain. We studied only African-American or white patients because of low prevalence (<5%) and heterogeneity of other races. Long-term Opioid Treatment Long-term opioid treatment was defined by three or more opioid prescriptions written at least 21 days apart within 6 months. For this determination and all analyses, we included oral, transdermal, or intranasal formulations of opioid medications except tramadol (not a controlled substance) and buprenorphine (not FDA-approved for treatment of chronic pain). Prescriptions that lacked data needed to calculate duration were excluded (i.e., start date, amount dispensed, or directions for use), as were prescriptions that were revised or reprinted within a 3-day window. The duration of opioid treatment, during which outcomes were assessed, lasted from the date of the first opioid prescription in the earliest 6-month period of long-term opioid treatment until 30 days after the final opioid prescription. Outcome Variables We created dichotomous outcome variables for each of three risk reduction strategies: 1) urine drug testing, 2) regular office visits, and 3) restricted early refills. Definitions of these variables were based on the minimal frequencies specified by guidelines and previous studies of these strategies. Urine drug testing was defined as at least one completed urine drug test for controlled substances at any point during opioid treat ment.7,8,24 Regular office visits was defined as at least one primary care visit (a) within each 6 months on long-term opioid treatment, and (b) within 30 days before or after each dose increase or change in opioid medication.8,9 To identify early refills of opioid prescriptions, we calculated the duration of a prescription based on the number dispensed and the directions, conservatively assuming that the patient took the medication at the maximal prescribed rate. We defined an early refill as being written at least 7 days before the previous prescription for the same medication should have been finished. Our definition of restricted early refills permitted up to one early refill as in previous studies.17 Classification of Risk Factors for Opioid Misuse Our main independent variables were five risk factors for opioid misuse derived from prior research: (1) age <45 at the time of the first opioid prescription,14–17,19 (2) current or past drug use disorder,14,15,17,20 (3) current or past alcohol use disorder,14,21 (4) current or past tobacco use,21,25 and (5) current or past mental health disorder (i.e., anxiety, depression, bipolar disorder, post-traumatic stress disorder, or schizophrenia).15,20–22 These conditions were identified from ICD-9-CM coded diagnoses from inpatient and outpatient visits (available online). Drug and alcohol use disorders were defined by an ICD-9-CM code for abuse, dependence, poisoning, withdrawal, or psychosis within our study timeframe. When the drug involved was an opioid, the diagnosis had to be made prior to the first opioid prescription because we wanted to identify risk factors for prescription opioid misuse. Codes for tobacco use and mental health disorders could occur at any time during the study timeframe. We examined each risk factor separately and analyzed an ordinal variable for the number of each patient’s risk factors, categorized into four groups: none, 1, 2, or 3 or more (3+). Other Covariates To adjust for potential patient confounders, we created sociodemographic, clinical, and health care variables. Sociodemographic variables included: sex, self-reported race, and median household income of the zip code of residence, based on U.S. census data.26 Clinical variables were defined from coded diagnoses for pain-related conditions and a medical comorbidity score developed following the approach of Elixhauser et al.27 Health care variables included: proportion of scheduled primary care appointments kept, as a measure of adherence to care;28 duration of opioid treatment; and total number of opioid prescriptions during the study timeframe. Because utilization of primary care services may influence receipt of urine drug testing or early refills, we created a three-level categorical variable to characterize utilization: (1) “high” if a patient visited at least once within each 6-month period of long-term opioid treatment and was in the highest quartile of monthly office visits; (2) “low” if a patient had at least one 6month period of opioid treatment without a visit and was in the lowest quartile of monthly office visits; and (3) “intermediate” for the remaining patients. For each patient, the usual source of primary care was based on the most frequently seen 960 Starrels et al.: Opioid Risk Reduction Strategies in Primary Care JGIM physician during the study timeframe and that physician’s main practice site. Statistical Analysis To examine the association of risk factors for opioid misuse with each of the three outcome variables of risk reduction strategies, we first examined bivariate associations using chi- square tests for categorical variables and the Cochran Armitage test of trend29 for the number of risk factors, an ordinal variable (none, 1, 2, or 3+). To adjust for clustering of patients within physicians, we conducted multivariate analyses using non-linear mixed effect regression models for each outcome, with physician as a random effect. We constructed 3 models for each outcome: the first included each known risk factor individually, the second included variables for each of the risk factor groups (1, 2, or 3+) with none as a referent group, and the third considered the ordinal variable (none, 1, 2, 3+) as continuous, to identify a linear relationship (trend) between the number of risk factors and the outcomes. Because the scant literature on this topic does not support the use of specific weights for the contribution of each risk factor to a patient’s overall risk for opioid misuse, we examined each risk factor individually as well as together, using the number of each patient’s risk factors as a proxy for global risk. To account for differences in the amount of time that patients were eligible for the outcomes, we adjusted for the duration of opioid treatment (weeks) in all models. We also adjusted for patient characteristics described previously: race, gender, median household income of zip code, appointment attendance rate, medical comorbidity score, and practice site. For analyses of the urine drug testing and restricted early refills outcomes, we included the primary care utilization variable to account for differences in opportunity to have these events. In post hoc analysis of restricted early refills, we examined the Pearson correlation of the number of early refills received by patients in relation to their total number of opioid prescriptions, and performed two sample t-tests to identify differences in the mean number of opioid prescriptions among patients with and without risk factors. Because drug use disorder is the risk factor for opioid misuse with the strongest evidence in the scientific literature, we conducted a sensitivity analysis incorporating a hierarchical risk scale that placed the highest value on this factor. 4057 patients with 3 opioid prescriptions (1/1/2004 - 12/31/2007) Figure 1. Study flow diagram. This analysis did not change our reported associations (available online). We also examined whether our results differed for patients who had been treated very long-term with opioids (i.e., 18 months or longer). In an unadjusted analysis, the relationship between the number of risk factors Table 1. Patient Characteristics Characteristic n=1612 Age, mean (SD) 54.1 (15.5) range, in whole years 19 to 94 Female, n (%) 1070 (66.4) Race, n (%) African-American 1001 (62.1) White 611 (37.9) Household income in neighborhood†, $, n (%) <25,000 407 (25.3) 25,000 – 34,999 546 (33.9) 35,000 – 69,999 544 (33.8) =70,000 115 (7.1) Pain-related diagnosis‡, n (%) Lumbago 830 (51.5) Osteoarthritis 693 (43.0) Neuropathic pain 427 (26.5) Cervical/thoracic spine pain 390 (24.2) Sciatica 375 (23.3) Fibromyalgia 260 (16.1) Rheumatoid arthritis 130 (8.1) Medical comorbidities §, n (%) None, n (%) 250 (15.5) 1, n (%) 412 (25.6) 2, n (%) 385 (23.9) 3+, n (%) 565 (35.1) Attendance to scheduled appointments mean (SD) 0.65 (0.15) range 0.21 to 1.00 Primary care utilization ¦, n (%) Low 256 (15.9) Intermediate 1008 (62.5) High 348 (21.6) Referred to pain specialist 251 (15.6) Referred to psychiatrist 99 (6.1) Duration on opioid treatment, in weeks Mean (SD) 100.0 (62.9) Range 10.3 to 219.9 Number of opioid prescriptions Mean (SD) 20.2 (22.9) Range 3 to 213 Opioid misuse risk factors Age<45, n (%) 469 (29.1) Drug use disorder, n (%) 123 (7.6) Alcohol use disorder, n (%) 73 (4.5) Tobacco use, n (%) 259 (16.1) Mental health disorder, n (%) 780 (48.8) Number of opioid misuse risk factors None, n (%) 533 (33.1) One, n (%) 614 (38.1) Two, n (%) 330 (20.4) Three or more, n (%) 135 (8.4) * Chi-square for categorical variables; t-test for continuous variables † At the zip-code level, using U.S. census data from 2000 ‡ Based on provider ICD-9-CM coding of inpatient and outpatient visits. Patients may have multiple pain diagnoses § Based on Elixhauser diagnoses as defined in Comorbidity Software version 3.4 (2009) ¦ “High” if a patient visited at least once within each 6-month period of long-term opioid treatment and was in the highest quartile of monthly office visits; “low” if a patient had at least one 6-month period of treatment without a visit and was in the lowest quartile of monthly office visits; and “intermediate” for the remaining patients Reasons for Exclusion: <3 qualifying prescriptions in 6 months (n = 1930) Cancer (n = 287) No pain diagnosis (n = 126) Missing race data (n= 78) Not African-American or white (n = 19) Age <18 (n= 5) 1612 patients included in analysis JGIM Starrels et al.: Opioid Risk Reduction Strategies in Primary Care 961 Table 2. Receipt of Opioid Risk Reduction Strategies by Risk Factors for Opioid Misuse Risk N Urine Drug Regular Office Restricted Early factor Test, Percent Visits, Percent Refills, Percent Total sample 1612 8.0 49.8 76.7 Age <45 469 11.5 45.2 74.6 =45 1143 6.6 51.7 77.4 p-value* <0.001 0.018 0.23 Drug use disorder Yes 123 29.3 52.9 66.7 No 1489 6.3 49.6 77.4 p-value* <0.001 0.48 0.01 Alcohol use disorder Yes 73 19.2 49.3 76.7 No 1539 7.5 49.8 76.6 p-value* <0.001 0.93 0.98 Tobacco use disorder Yes 259 14.7 48.3 72.2 No 1353 6.7 50.1 77.5 p-value* <0.001 0.59 0.067 Mental health disorder Yes 780 11.3 48.9 74 No 832 4.9 50.6 79.1 p-value* <0.001 0.5139 0.0154 * Chi-square test for misuse and each outcome in this group did not differ from those observed for full sample and are therefore not presented. RESULTS The study cohort included 1,612 primary care patients who received long-term opioid treatment for CNCP (Figure 1). Most study patients were female, African-American, and resided in zip codes where median household income was below $35,000 per year (Table 1). The prevalence of risk factors for opioid misuse was as follows: age<45 (29.1%), drug use disorder (7.6%), alcohol use disorder (4.5%), tobacco use (16.1%), and mental health disorder (48.4%). The mean duration of observed opioid treatment was 1.9 years, and 54.5% of the cohort was treated for at least 3.0 50.7 80.1 6.2 51.6 77.7 12.7 47.0 71.2 24.4 45.2 71.1 0 10 20 30 40 50 60 70 80 90 Urine Drug Testing* Regular Office Visits† Restricted Early Refills‡ Percent 0 1 2 3+ Cochran Armitage test of trend, p<0.001 †Cochran Armitage test of trend, p=0.16 ‡Cochran Armitage test of trend, p=0.001 Figure 2. Receipt of recommended opioid risk management practices, by number of patient risk factors for opioid misuse. 18 months. During this time, patients received a mean of 20.2 opioid prescriptions (SD 22.9). Within the study cohort, 8.0% had at least one urine drug test, 49.8% had regular office visits, and 76.6% had restricted early refills. Regarding the two criteria for having regular office visits, 61.0% of the cohort had at least one visit within every 6-month period on opioids, and 75.3% had a visit within 30 days of every opioid medication change. Overall, 44 (2.7%) of the cohort received all three risk reduction strategies. Urine Drug Testing. Before adjustment, urine drug testing was significantly associated with each of the five risk factors for opioid misuse (Table 2). A drug use disorder was associated with over a fourfold increase in the unadjusted odds of urine drug testing, and after adjustment, the odds were still increased by threefold (Table 3). Both unadjusted and adjusted odds of urine drug testing were significantly increased by approximately twofold for a mental health disorder. Although urine drug testing increased with an increasing number of risk factors for opioid misuse (test of trend, p<0.001), only 24.4% of the highest-risk patients (i.e., three or more risk factors) received any urine drug testing Table 3. Association of Opioid Misuse Risk Factors with Urine Drug Testing Unadjusted Odds Ratio 95% CI P-value Adjusted Odds Ratio* 95% CI P-value Opioid misuse risk factors Age <45 1.65 1.13-2.42 0.01 1.41 0.92-2.15 0.12 Drug use disorder 4.46 2.78-7.13 <0.001 3.18 1.94-5.21 <0.001 Alcohol use disorder 1.60 0.82-3.13 0.17 1.35 0.67-2.65 0.40 Tobacco use 1.58 1.02-2.44 0.04 1.37 0.88-2.14 0.16 Mental health disorder 1.98 1.33-2.95 0.001 1.73 1.14-2.65 0.01 No. of opioid misuse risk factors 0 1.0 N/A N/A 1.00 N/A N/A 1 2.13 1.18-3.87 0.01 1.79 0.97-3.29 0.06 2 4.71 2.60-8.53 <0.001 3.10 1.66-5.81 <0.001 3+ 10.54 5.55-19.7 <0.001 6.08 3.11-11.87 <0.001 Trend† <0.001 <0.001 * Adjusted for race, gender, median household income of zip code, duration of time on opioids, appointment attendance rate, medical comorbidity score, utilization category, and practice site. Models of individual opioid misuse risk factors adjusted for the other four risk factors † In unadjusted analyses, using the Cochran Armitage test of trend. In adjusted analyses, using nonlinear mixed effect models and the number of risk factors (none, 1, 2, and 3+) as a continuous variable 962 Starrels et al.: Opioid Risk Reduction Strategies in Primary Care JGIM Table 4. Association of Opioid Misuse Risk Factors with Regular Office Visits Unadjusted Odds Ratio 95% CI P-value Adjusted Odds Ratio* 95% CI P-value Opioid misuse risk factors Age <45 0.77 0.62-0.96 0.02 0.80 0.61-1.06 0.12 Drug use disorder 1.21 0.83-1.78 0.33 1.16 0.73-1.82 0.54 Alcohol use disorder 0.97 0.60-1.57 0.91 0.81 0.46-1.44 0.47 Tobacco use 0.94 0.72-1.24 0.66 0.79 0.57-1.10 0.17 Mental health disorder 0.95 0.78-1.15 0.59 1.01 0.79-1.29 0.94 No. of opioid misuse risk factors 0 1.0 N/A N/A 1.00 N/A 1 1.04 0.82-1.31 0.74 1.05 0.79-1.40 0.74 2 0.86 0.66-1.14 0.29 0.96 0.68-1.36 0.81 3+ 0.80 0.55-1.17 0.26 0.69 0.43-1.11 0.12 Trend† 0.16 0.20 * Adjusted for race, gender, median household income of zip code, duration of time on opioids, appointment attendance rate, medical comorbidity score, and practice site. Models of individual opioid misuse risk factors adjusted for the other four risk factors † In unadjusted analyses, using the Cochran Armitage test of trend. In adjusted analyses, using nonlinear mixed effect models and the number of risk factors (none, 1, 2, and 3+) as a continuous variable (Figure 2). In fully adjusted models, the odds of urine drug testing were increased threefold for patients with two risk factors and sixfold for patients with three or more risk factors, compared with no risk factors (Table 3). Regular Office Visits. Before adjustment, regular office visits were less likely for patients under 45 years old (Table 2). However, after adjustment, making regular office visits was no longer associated with any risk factor or with the number of risk factors (test of trend, p=0.20) (Table 4). Restricted Early Refills. Patients with a drug use or mental health disorder were significantly more likely to receive >1 early refill (less likely to have restricted early refills) (Table 2). After full adjustment, the odds of restricted early refills were significantly reduced by over 40% for a drug use disorder (Table 5), but no other risk factor was associated with this outcome. The number of patient risk factors was also inversely related to restricted early refills (p=0.001), with a 36% reduction in the adjusted odds of restricted early refills for patients with two risk factors and a 44% reduction for those with at least three risk factors, compared with no risk factors. The number of early refills was highly correlated with the patient’s total number of opioid prescriptions during the study time frame (r=0.72). Patients with a drug use disorder received a greater number of opioid prescriptions than patients without a drug use disorder (mean of 27.5 and 19.6 prescriptions, respectively, p<0.001), and received more prescriptions per month on opioid treatment (mean of 1.11 and 0.82, respectively, p<0.001), though their duration on opioid treatment was not significantly longer (mean of 103.3 and 99.7 weeks, respectively, p=0.728). DISCUSSION Among over 1600 primary care patients prescribed long- term opioids for CNCP, monitoring with recommended risk reduction strategies was quite limited. While being treated with long-term opioids, fewer than 10% of the cohort received any urine drug testing, only half had regular office based visits, and 23% received more than one early opioid refill. Although patients at increased risk of opioid misuse were more likely to have had urine drug testing, it was still infrequent, with less than one-quarter of the patients with three or more risk factors having any urine drug test. In addition, patients at increased risk of opioid misuse were more likely to receive more than one early refill, but their office-based Table 5. Association of Opioid Misuse Risk Factors with Restricted Early Refills Unadjusted Odds Ratio 95% CI P-value Adjusted Odds Ratio* 95% CI P-value Opioid misuse risk factors Age <45 0.89 0.71-1.15 0.39 0.83 0.60-1.13 0.24 Drug use disorder 0.63 0.42-0.95 0.03 0.56 0.34-0.92 0.02 Alcohol use disorder 1.22 0.69-2.16 0.50 1.04 0.54-2.01 0.91 Tobacco use 0.84 0.61-1.14 0.26 0.79 0.55-1.14 0.21 Mental health disorder 0.79 0.63-1.00 0.05 0.95 0.72-1.26 0.73 No. of opioid misuse risk factors 0 1.0 N/A 1.00 N/A 1 0.86 0.65-1.15 0.32 0.85 0.61-1.19 0.35 2 0.61 0.45-0.85 0.003 0.64 0.43-0.96 0.03 3+ 0.61 0.40-0.94 0.02 0.56 0.33-0.95 0.03 Trend† <0.001 0.008 * Adjusted for race, gender, median household income of zip code, duration of time on opioids, appointment attendance rate, medical comorbidity score, utilization category, and practice site. Models of individual opioid misuse risk factors adjusted for the other four risk factors † In unadjusted analyses, using the Cochran Armitage test of trend. In adjusted analyses, using nonlinear mixed effect models and the number of risk factors (none, 1, 2, and 3+) as a continuous variable JGIM Starrels et al.: Opioid Risk Reduction Strategies in Primary Care 963 monitoring was no greater than for persons without any risk factors for opioid misuse. These data reveal limited monitoring of all study patients on long-term opioids and this lax monitoring extends even to patients at greater risk of misuse. Previous studies have demonstrated limited use of opioid risk reduction strategies in primary care settings, but none has evaluated the association with opioid misuse risk factors.24,30–34 In two primary care studies, only 2 to 18% of patients received urine drug testing.24,30 We found a similar overall low rate (8%) of urine drug testing but, despite a three to sixfold increase in the adjusted odds of urine testing for patients with multiple risk factors for misuse, only a minority were tested. Reasons for limited urine drug testing by primary care physicians might include lack of knowledge about the tests,35,36 concerns that patients will feel stigmatized,37 and a limited evidence base.11 However, urine drug testing performs better in identifying misuse than clinician judgment38 or patient self-report.39,40 Physicians who monitor with urine drug tests report enhanced communication with their patients and improved safety of prescribed opioids.41–43 To our knowledge, this is the first analysis of the frequency of primarycareofficevisitsduringopioidtreatment. Surprisingly, only half of patients met this minimum recommended frequency of office-based monitoring of patients on long-term opioid therapy.8 Furthermore, patients at higher risk of opioid misuse were not seen more regularly in the office. This lack of face-toface encounters represents missed opportunities for physicians to examine responses to treatment, propose alternative treatments when response is inadequate, detect side effects, and assess for misuse. In previous studies, the proportion of patients receiving early opioid refills ranged from 4% to 41%,17,18,44 and 15% in a primary care setting.17 We found that 23% of our cohort received more than one early opioid refill. But of even greater concern, patients with a current or past drug use disorder were more likely to receive early refills. This may be due to more requests for early refills from patients with a drug use disorder.18 Indeed, these patients averaged more prescriptions per month than patients without a drug use disorder, consistent with prior observations.45 Physicians providing these early refills may be unwittingly contributing to misuse or diversion. Requests for early refills among patients with a drug use disorder may also reflect inadequate analgesia, possibly because of greater analgesic requirements,46 or because cautious physicians may restrict the dose of opioids. We acknowledge several limitations to this study. First, we studied 8 practices in a single university health system, which limits generalizability. Second, our clinical information comes from an electronic medical record and there may be misclassification, especially for drug and alcohol use disorders, as well as unmeasured confounding that could influence documentation of risk factors. Third, our selection of risk factors was based on observational studies of varying quality. Fourth, we could not account for patient differences in the severity of each risk factor. Fifth, our outcome variables were based on expert consensus but lack evidence of their impact on safety.11 Finally, the timeframe in which we assessed the presence of opioid misuse risk factors and risk reduction strategies overlapped. Therefore, we cannot determine whether and when the physician became aware of a patient’s risk factors, or whether the diagnosis of a risk factor such as drug use disorder was made prior to, or as a result of, a risk reduction strategy being performed. Overall, our findings reveal disturbingly low use of monitoring strategies to reduce the risk of adverse events from long-term opioid treatment and indicate that primary care physicians are not employing all these approaches more intensively for patients at increased risk of misuse. Our study supports recommendations for a more standardized approach to opioid risk reduction. 11,13 A standardized approach could involve using screening tools to identify patients at increased risk for opioid 25,47–50 misuse; a treatment agreement that stipulates the necessity for regular office visits, restricted early refills, and urine drug testing;51 and team-based care to track patients’ visits, prescriptions, progress, and aberrant drug-taking behaviors. 52 Our research demonstrates the need for proactive approaches to this quality of care issue that has significant public health consequences. Acknowledgements: This work was supported through the Program of Research Integrating Substance Use Issues into Mainstream Health Care (PRISM), funded by the Robert Wood Johnson Foundation, the National Institute on Drug Abuse (NIDA), and the Substance Abuse and Mental Health Services Administration (SAMHSA). In addition, Dr. Starrels was supported in part by the Robert Wood Johnson Foundation Clinical Scholars Program and the Office of Veterans Affairs as a VA Special Fellow. The authors thank Evelyn Crowley, MS for contributing to data management and the Montefiore Division of General Medicine Substance Abuse Research Group for feedback during preparation of the manuscript. Preliminary results of this study were presented at the 32nd annual meeting of the Society of General Internal Medicine, Miami Beach, Florida, 13-16 May 2009. Conflict of Interest: None disclosed. Corresponding Author: Joanna L. Starrels, MD, MS; Division of General Internal Medicine, Albert Einstein College of Medicine and Montefiore Medical Center, 111 E. 210th Street, Bronx, NY 10467, USA (e-mail: jostarre@montefiore.org). REFERENCES 1. Substance Abuse and Mental Health Services Administration, Office of Applied Studies. The NSDUH Report: Patterns and trends in nonmedical prescription pain reliever use: 2002 to 2005. Rockland, MD.; (April 6, 2007): Accessed on January 20, 2011. Available from: http://oas. samhsa.gov/2k7/pain/pain.pdf 2. Substance Abuse and Mental Health Services Administration, Office of Applied Studies. Treatment Episode Data Set (TEDS) Highlights– 2006 National Admissions to Substance Abuse Treatment Services. OAS Series #S-40, DHHS Publication No. (SMA) 08-4313, Rockville, MD, 2007. 3. Centers for Disease Control and Prevention. Unintentional Poisoning Deaths– United States, 1999-2004. MMWR Morb Mortal Wkly Rep [serial on the Internet]. 56(5): Available from: Accessed on January 20 2011. Available from: http://www.cdc.gov/mmwr/preview/mmwrhtml/ mm5605a1.htm. 4. Paulozzi LJ, Budnitz DS, Xi Y. Increasing deaths from opioid analgesics in the United States. Pharmacoepidemiol Drug Saf. 2006;15(9):618–27. 5. Caudill-Slosberg MA, Schwartz LM, Woloshin S. Office visits and analgesic prescriptions for musculoskeletal pain in US: 1980 vs. 2000. Pain. 2004;109(3):514–9. 6. The use of opioids for the treatment of chronic pain. A consensus statement from the American Academy of Pain Medicine and the American Pain Society. Clin J Pain. 1997;13:6–8. 7. Federation of State Medical Boards of the United States. Model policy for the use of controlled substances for the treatment of pain. 2004. 8. Chou R, Fanciullo GJ, Fine PG, Adler JA, Ballantyne JC, Davies P, Donovan MI, Fishbain DA, Foley KM, Fudin J, Gilson AM, Kelter A, Mauskop A, O'Connor PG, Passik SD, Pasternak GW, Portenoy RK, Rich BA, Roberts RG, Todd KH, Miaskowski C. Clinical guidelines for the use of chronic opioid therapy in chronic noncancer pain. J Pain. 2009;10(2):113–30. 964 Starrels et al.: Opioid Risk Reduction Strategies in Primary Care JGIM 9. Sundwall DN, Rolfs RT, Johnson E. Utah Department of Health. Utah Clinical Guidelines of Prescribing Opioids for Treatment of Chronic Pain (2009). Salt Lake City, UT. Accessed January 20 2011. Available from: http:// www.dopl.utah.gov/licensing/forms/OpioidGuidlines_summary.pdf. 10. Denisco RA, Chandler RK, Compton WM. Addressing the intersecting problems of opioid misuse and chronic pain treatment. Exp Clin Psychopharmacol. 2008;16(5):417–28. 11. Starrels JL, Becker WC, Alford DA, Williams AR, Kapoor A, Turner BJ. Opioid treatment agreements and urine drug testing in chronic pain: a systematic review. Ann Intern Med. 2010;152:712–20. 12. Chou R, Ballantyne JC, Fanciullo GJ, Fine PG, Miaskowski C. Research gaps on use of opioids for chronic noncancer pain: findings from a review of the evidence for an American Pain Society and American Academy of Pain Medicine clinical practice guideline. J Pain. 2009;10(2):147–59. 13. Gourlay DL, Heit HA, Almahrezi A. Universal precautions in pain medicine: A rational approach to the treatment of chronic pain. Pain Med. 2005;6(2):107–12. 14. Ives TJ, Chelminski PR, Hammett-Stabler CA, Malone RM, Perhac JS, Potisek NM, Shilliday BB, DeWalt DA, Pignone MP. Predictors of opioid misuse in patients with chronic pain: a prospective cohort study. BMC Health Serv Res. 2006;6:46. 15. Edlund MJ, Steffick D, Hudson T, Harris KM, Sullivan M. Risk factors for clinically recognized opioid abuse and dependence among veterans using opioids for chronic non-cancer pain. Pain. 2007;129(3):355–62. 16. Michna E, Jamison RN, Pham L-D, Ross EL, Janfaza D, Nedeljkovic SS, Narang S, Palombi D, Wasan AD. Urine toxicology screening among chronic pain patients on opioid therapy: frequency and predictability of abnormal findings. Clin J Pain. 2007;23(2):173–9. 17. Reid MC, Engles-Horton LL, Weber MB, Kerns RD, Rogers EL, O'Connor PG. Use of opioid medications for chronic noncancer pain syndromes in primary care. J Gen Intern Med. 2002;17(3):173–9. 18. White AG, Birnbaum HG, Schiller M, Tang J, Katz NP. Analytic models to identify patients at risk for prescription opioid abuse. Am J Manag Care. 2009;15(12):897–906. 19. Hall AJ, Logan JE, Toblin RL, Kaplan JA, Kraner JC, Bixler D, Crosby AE, Paulozzi LJ. Patterns of abuse among unintentional pharmaceutical overdose fatalities. JAMA. 2008;300(22):2613–20. 20. Morasco BJ, Dobscha SK. Prescription medication misuse and substance use disorder in VA primary care patients with chronic pain. Gen Hosp Psychiatry. 2008;30(2):93–9. 21. Michna E, Ross EL, Hynes WL, Nedeljkovic SS, Soumekh S, Janfaza D, Palombi D, Jamison RN. Predicting aberrant drug behavior in patients treated for chronic pain: importance of abuse history. J Pain Symptom Manage. 2004;28(3):250–8. 22. Wasan AD, Butler SF, Budman SH, Benoit C, Fernandez K, Jamison RN. Psychiatric history and psychologic adjustment as risk factors for aberrant drug-related behavior among patients with chronic pain. Clin J Pain. 2007;23(4):307–15. 23. Dunn KM, Saunders KW, Rutter CM, Banta-Green CJ, Merrill JO, Sullivan MD, Weisner CM, Silverberg MJ, Campbell CI, Psaty BM, Von Korff M. Opioid prescriptions for chronic pain and overdose: a cohort study. Ann Intern Med. 2010;152(2):85–92. 24. Yanni LM, Weaver MF, Johnson BA, Morgan LA, Harrington SE, Ketchum JM. Management of chronic nonmalignant pain: a needs assessment in an internal medicine resident continuity clinic. J Opioid Manag. 2008;4(4):201–11. 25. Akbik H, Butler SF, Budman SH, Fernandez K, Katz NP, Jamison RN. Validation and clinical application of the Screener and Opioid Assessment for Patients with Pain (SOAPP). J Pain Symptom Manage. 2006;32(3):287–93. 26. U.S. Census Bureau; Census 2000, Summary File 3; generated by Joanna Starrels; using American FactFinder >http://factfinder.census. gov/>; (11 June 2008) 27. Elixhauser A, Steiner C, Harris DR, Coffey RM. Comorbidity measures for use with administrative data. Med Care. 1998;36(1):8–27. 28. Turner BJ, Hollenbeak CS, Weiner M, Ten Have T, Tang SS. Effect of unrelated comorbid conditions on hypertension management. Ann Intern Med. 2008;148(8):578–86. 29. Agresti A. Categorical Data Analysis, 2nd Edition. Hoboken, NJ: John Wiley & Sons, Inc; 2002. 30. Adams NJ, Plane MB, Fleming MF, Mundt MP, Saunders LA, Stauffacher EA. Opioids and the treatment of chronic pain in a primary care sample. J Pain Symptom Manage. 2001;22(3):791–6. 31. Bhamb B, Brown D, Hariharan J, Anderson J, Balousek S, Fleming MF. Survey of select practice behaviors by primary care physicians on the use of opioids for chronic pain. Curr Med Res Opin. 2006;22(9):1859–65. 32. Boulanger A, Clark AJ, Squire P, Cui E, Horbay GLA. Chronic pain in Canada: Have we improved our management of chronic noncancer pain? Pain Res Manage. 2007;12(1):39–47. 33. Touchet BK, Yates WR, Coon KA. Opioid contract use is associated with physician training level and practice specialty. J Opioid Manage. 2005;1 (4):195–200. 34. Watkins A, Wasmann S, Dodson L, Hayes M. An evaluation of the care provided to patients prescribed controlled substances for chronic nonmalignant pain at an academic family medicine center. Fam Med. 2004;36(7):487–9. 35. Reisfield GM, Webb FJ, Bertholf RL, Sloan PA, Wilson GR. Family physicians' proficiency in urine drug test interpretation. J Opioid Manage. 2007;3(6). 36. Tellioglu T. The use of urine drug testing to monitor patients receiving chronic opioid therapy for persistent pain conditions. Med Health R I. 2008;91(9):279–80. 82. 37. Swanson J. Urine drug screening for opioids. J Pain Palliat Care Pharmacother. 2002;16(1):111–4. 38. Katz NP, Sherburne S, Beach M, Rose RJ, Vielguth J, Bradley J, Fanciullo GJ. Behavioral monitoring and urine toxicology testing in patients receiving long-term opioid therapy. Anesth Analg. 2003;97 (4):1097–102. 39. Fishbain DA, Cutler RB, Rosomoff HL, Rosomoff RS. Validity of self- reported drug use in chronic pain patients. Clin J Pain. 1999;15(3):184– 91. 40. Atluri S, Sudarshan G. Evaluation of abnormal urine drug screens among patients with chronic non-malignant pain treated with opioids. Pain Physician. 2003;6:407–9. 41. Heit HA, Gourlay DL. Urine drug testing in pain medicine. J Pain Symptom Manage. 2004;27(3):260–7. 42. Sampson JM, Achololnu WW Jr. Reducing patient aggression and hostility in primary care with urine drug testing. South Med J. 2004;97 (9):916–7. 43. Institute for Clinical Systems Improvement. Assessment and management of chronic pain: percentage of patients diagnosed with chronic pain who are prescribed an opioid who have an opioid agreement form and urine toxicology screen documented in the medical record (July 2008). Bloomington, MN.: Accessed on January 20 2011. Available from: http://www.qualitymeasures.ahrq.gov/summary/summary.aspx?doc_ id=13005&string=urine±AND±pain. 44. Fleming MF, Balousek SL, Klessig CL, Mundt MP, Brown DD. Substance use disorders in a primary care sample receiving daily opioid therapy. J Pain. 2007;8(7):573–82. 45. Weisner CM, Campbell CI, Ray GT, Saunders K, Merrill JO, Banta-Green C, Sullivan MD, Silverberg MJ, Mertens JR, Boudreau D, Von Korff M. Trends in prescribed opioid therapy for non-cancer pain for individuals with prior substance use disorders. Pain. 2009;145(3):287–93. 46. Savage SR, Kirsh KL, Passik SD. Challenges in using opioids to treat pain in persons with substance use disorders. Addict Sci Clin Pract. 2008;4(2):4–25. 47. Compton PA, Wu SM, Schieffer B, Pham Q, Naliboff BD. Introduction of a self-report version of the Prescription Drug Use Questionnaire and relationship to medication agreement noncompliance. J Pain Symptom Manage. 2008;36(4):383–95. 48. Webster LR, Webster RM. Predicting aberrant behaviors in opioid- treated patients: preliminary validation of the Opioid Risk Tool. Pain Med. 2005;6(6):432–42. Nov-Dec. 49. Holmes CP, Gatchel RJ, Adams LL, Stowell AW, Hatten A, Noe C, Lou L. An opioid screening instrument: long-term evaluation of the utility of the Pain Medication Questionnaire. Pain Pract. 2006;6(2):74–88. 50. Belgrade MJ, Schamber CD, Lindgren BR. The DIRE Score: Predicting Outcomes of Opioid Prescribing for Chronic Pain. J Pain. 2006;7(9):671– 81. 51. Fishman SM, Bandman TB, Edwards A, Borsook D. The opioid contract in the management of chronic pain. J Pain Symptom Manage. 1999;18(1):27–37. 52. Wiedemer NL, Harden PS, Arndt IO, Gallagher RM. The opioid renewal clinic: A primary care, managed approach to opioid therapy in chronic pain patients at risk for substance abuse. Pain Med. 2007;8(7):573–84.